The use of Artificial Intelligence for Idea Generation in the Innovation Process
Ode Plätke and Richard C. Geibel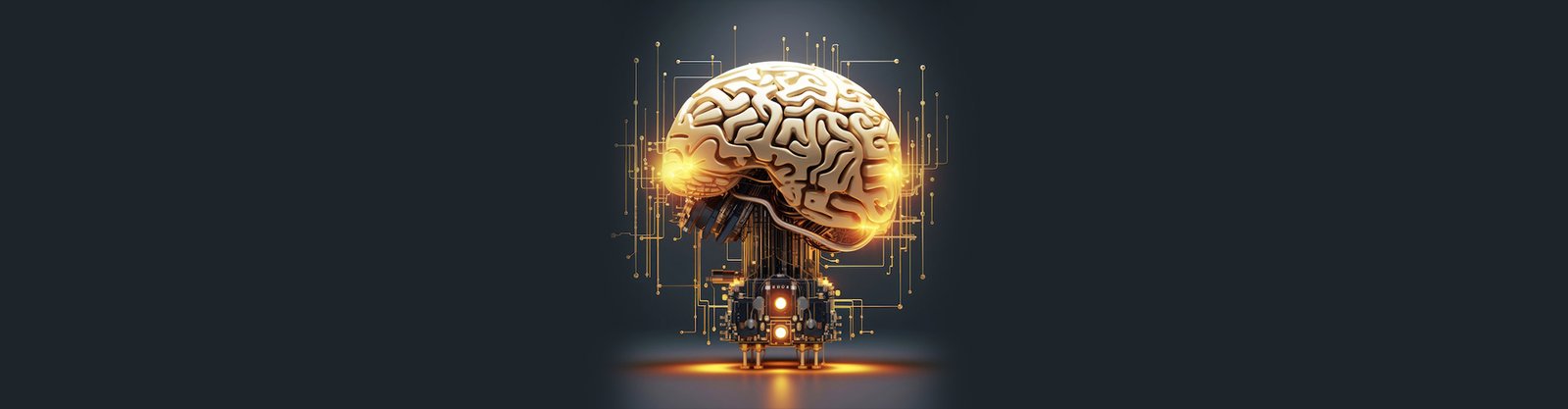
Introduction
Theory
Methodology
Results
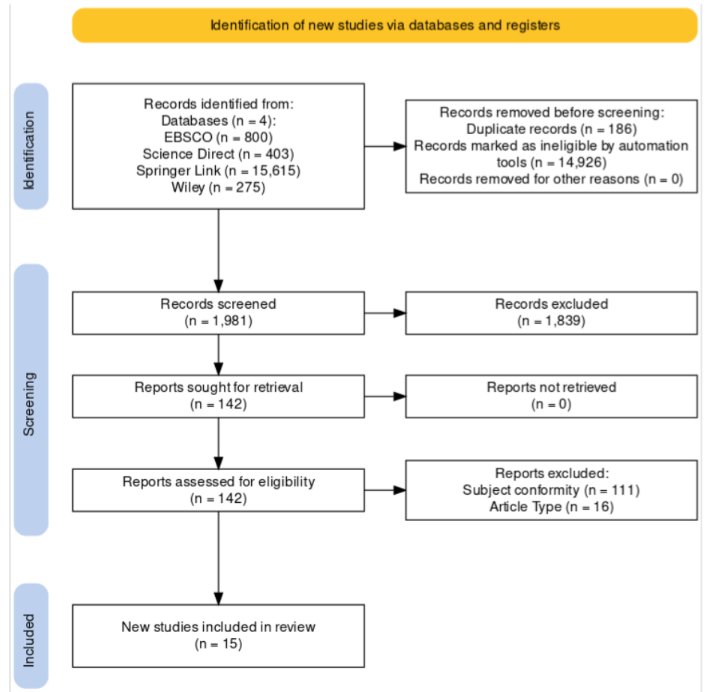
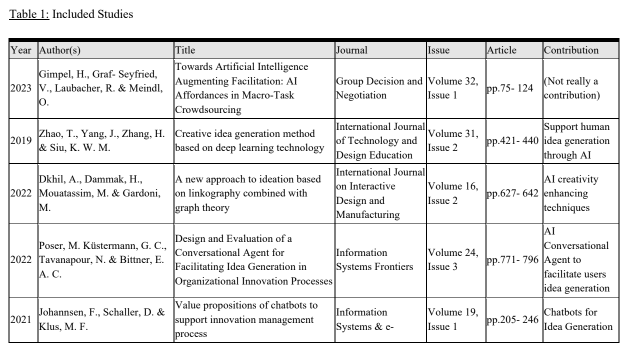
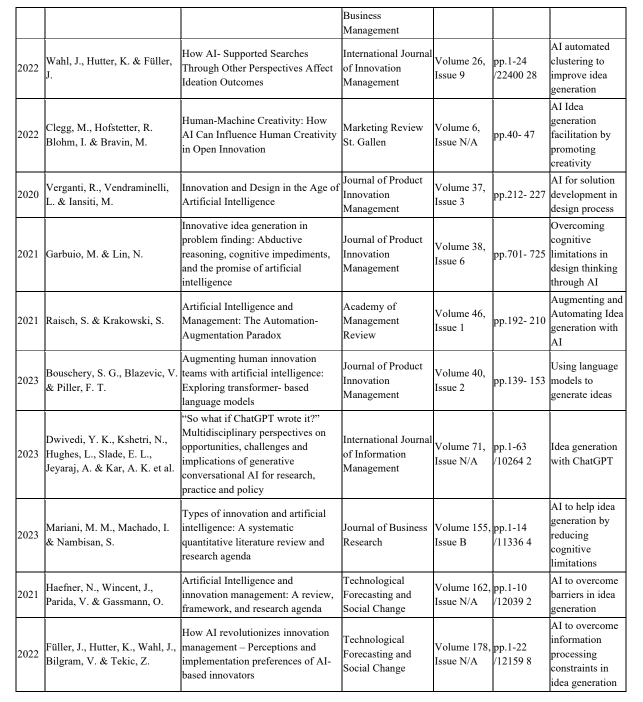
4.1 Risk of Bias Assessment
The Quality of each Article was assessed using a three-level rating system, represented by the following symbols:
(✓): The criterion is met, indicating low risk of bias or high methodological quality.
(?): It is unclear whether the criterion is met due to insufficient information, indicating unclear risk of bias or uncertain methodological quality.
(X): The criterion is not met, indicating a high risk of bias or low methodological quality.
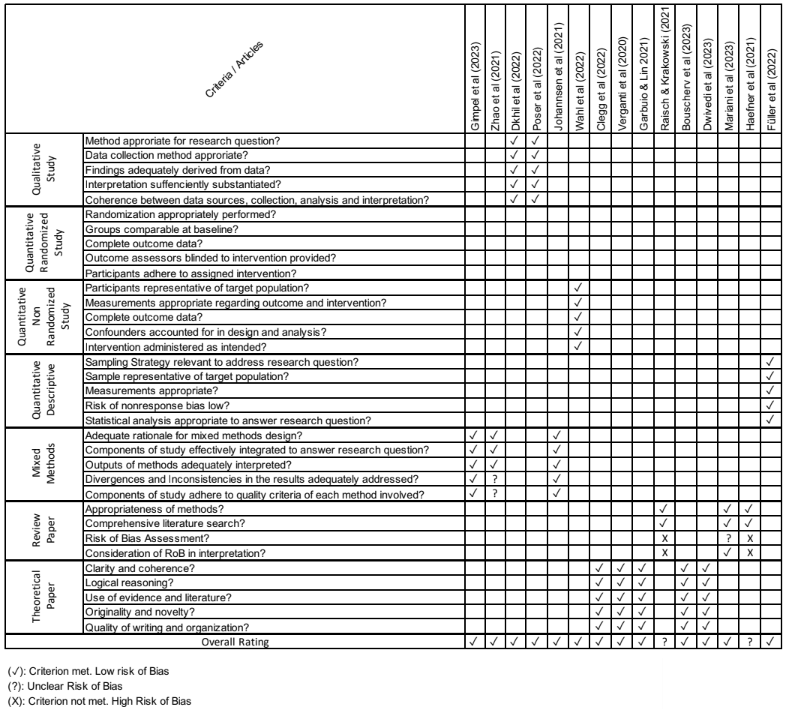
4.2 Thematic Synthesis of Applications
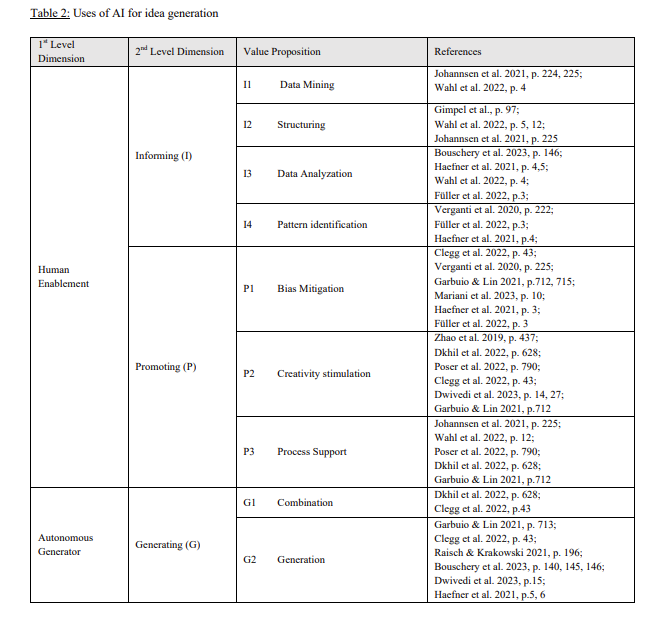
4.3 Thematic Synthesis of Limitations
4.3.1 Implementation
The first dimension, “Implementation”, comprises value propositions related to the practical challenges of applying AI in innovation management. These include “Application“, “Accessibility” and “Technology Readiness.”
- Under the "Application" value proposition, it is noted that AI-based innovation management does not lend itself to universal application. Instead, tailored approaches are required to address specific innovation challenges, rejecting the one-size-fits-all approach. This highlights the need for adaptive and flexible AI systems that can cater to unique contexts and requirements“
- "Accessibility" is another dimension where challenges are evident. Unstable internet connections are identified as a hindrance to the accessibility of AI, emphasizing the dependency on robust and stable infrastructure. This limitation brings attention to the digital divide, which could restrict the reach and effectiveness of AI in innovation management.
- The "Technology Readiness" value proposition points out that the technological capabilities of AI systems remain relatively limited, with few systems meeting the desired level of readiness. The gap between the theoretical potential of AI and its practical usability in real-world scenarios is underscored. The maturity of natural language processing mechanisms is also deemed insufficient for human-like conversations with chatbots, highlighting the preference for human communication partners.
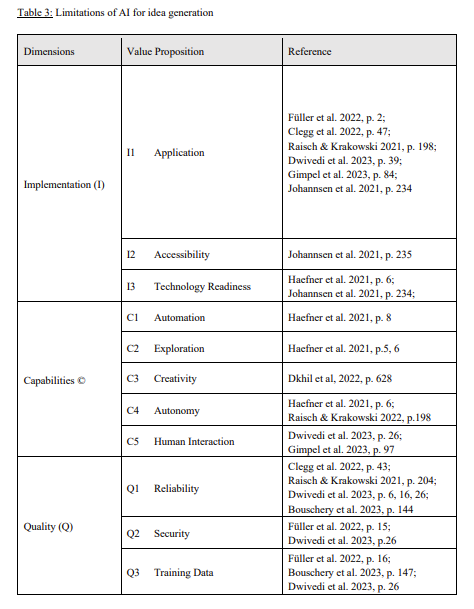
4.3.2 Capabilities
Moving to the second dimension, “Capabilities”, various value propositions shed light on AI’s limitations in performing specific functions or achieving desired outcomes. These include “Automation“, “Exploration“, “Creativity“, “Autonomy” and “Human Interaction.”
- Concerning "Automation", AI solutions aim to automate workflows, but it is recognized that an entire set of tasks cannot always be fully automated. This implies the need for human involvement and oversight in processes that are too complex or nuanced for AI to handle independently.
- In the domain of "Exploration", AI systems are noted to have limited ability to explore problem spaces, a crucial function often required in innovation management. The inability of AI to explore uncharted territories and acquire new knowledge presents a limitation in innovation contexts.
- The "Creativity" value proposition highlights that AI struggles to emulate human creativity, a central aspect of innovation management. Given the importance of creativity in innovation, this limitation is of particular significance“
- "Autonomy" refers to AI systems' capacity to operate independently. These systems often struggle to navigate solution spaces independently, lacking self-awareness and purposefulness. As a result, human involvement in specifying goals remains essential in innovation management.
- Under the "Human Interaction" value proposition, issues may arise in human-computer interaction. AI, such as ChatGPT, may struggle to understand certain nuances or contexts, and ethical and cultural factors may be challenging to grasp. These limitations point to potential shortcomings in AI's ability to fully mimic human interactions.
4.3.3 Quality
The third and final dimension, “Quality”, includes value propositions like “Reliability“, “Data Security” and “Training Data.”
- The "Reliability" value proposition raises concerns about the trustworthiness of AI systems and the quality of their results. It is noted that the quality and context of AI-generated ideas should be rigorously tested, as AI can introduce statistical biases and limitations. Understanding the results of AI, particularly those generated by pattern-based methods, can be challenging, emphasizing the need for human validation“
- "Data Security" is another critical dimension, where issues related to privacy, security, and potential misuse of data are highlighted. These concerns underscore the importance of addressing data security when using AI systems in innovation management.
- The "Training Data" value proposition underscores the significance of the data used to train AI systems. Insufficient labeled training data and data quality issues can hinder AI's effectiveness. Bias in training data can potentially harm language models. High-quality, representative datasets are deemed essential for optimal AI performance.
Conclusion
References
Bouschery, S. G., Blazevic, V. & Piller, F. T., 2023, ‘Augmenting human innovation teams with artificial intelligence: Exploring transformerbased language models’, Journal of Product Innovation Management, 40(2), 129-153